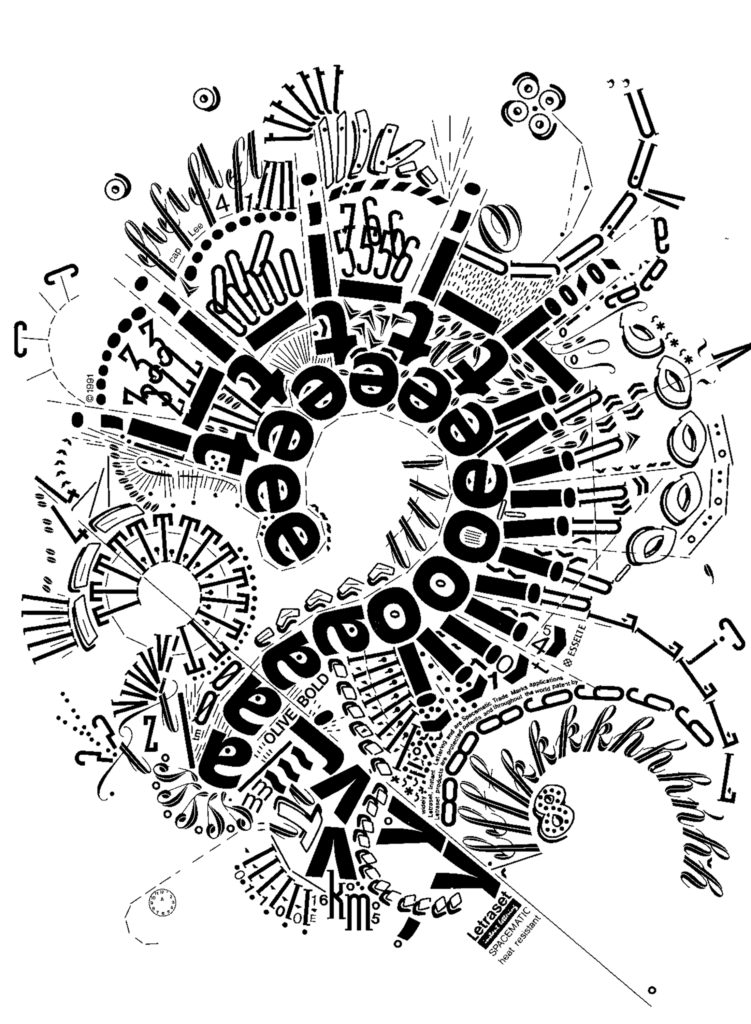
by Rachel Melzi
A friend wrote this report on her experience as a translator, questioning the general hype about artificial intelligence without denying the influence technology has on her work. Her report will be published in the German magazine Wildcat. We previously translated a more general article on AI by Wildcat, which can be found here.
As a freelance Italian to English translator, people increasingly ask me whether AI is going to make me redundant. While I should probably be outraged at the question, arguing that my creativity could never be replaced by a machine, in reality a lot of the texts I translate are of such poor quality that they probably could be translated by computers in the not too distant future. Before looking more closely at the impact of AI on translation, I will give a brief overview of my work in the context of translators worldwide and point to some other equally important ways in which digitalisation has affected translation.
Platforms and crowdsourcing
Unlike most translators, I translate and edit only academic articles in the arts and social sciences and deal directly with a small group of clients (largely Italian universities) who pay me per word on very short-term contracts. After five years doing this work, I now earn about the same per year as a new Italian secondary school teacher (around 23,000 euros) and as a freelancer pay 24% national insurance and 5%-15% tax. While my pay is below the global average for a translator (26,000 euros a year), it is above the median, with 49% of translators worldwide earning less than 18,000 euros and 21% less than 4500 euros. [1]
Across the world, the vast majority of translators say digital labour platforms are important for their work (85%). The jobs they get through these platforms tend to be from foreign agencies rather than direct or local clients. To give you an idea of their size, one of the biggest platforms, Proz, currently lists 31,000 English to Italian translators and 6400 Italian to English translators. Although no specific qualification is required to register, only a small minority are not university educated. Competition is such that translators using these platforms tend to work long hours for low pay: a recent study in Turkey for instance, shows 44% of translators frequently worked over 48 hours a week and 77% earnt below the union defined “poverty line”. [2]
At some point I might also be forced onto an Italian government online platform called MePA, in which users bid against each other to do temporary jobs in the public sector. Initially designed for institutions to buy or rent commodities (e.g. renting chairs for an event) it is now widely used for services such as academic translation. The system favours agencies over individual translators, and of course favours the lowest quote. Increasing numbers of universities seem to be using MePA for all their translation work, and so once the universities I work with do the same, my income is likely to fall.
Another downward pressure on wages comes from the “crowdsourcing” of translations – basically free translations in the name of some undefined good – used by companies like Facebook and X, and even by translation platforms themselves. This is reinforced by organisations such as Translators Without Borders, which gets people to volunteer to translate for humanitarian causes and then exploits their translations for commercial purposes. [3]
Although it would be interesting to further explore the effect of digital platforms and of “volunteer” translators, the rest of this article will focus only on the technicalities of AI translation and whether it could at some point replace human translators.
How good is AI translation?
Already in 2020, two thirds of professional translators used “Computer-assisted translation” or CAT (CSA Research, 2020). Whereas “machine translation” translates whole documents, and thus is meant to replace human translation, CAT supports it: the computer makes suggestions on how to translate words and phrases as the user proceeds through the original text. The software can also remind users how they have translated a particular word or phrase in the past, or can be trained in a specific technical language, for instance, by feeding it legal or medical texts. CAT software is currently based on Neural Machine Translation (NMT) models, which are trained through bilingual text data to recognise patterns across different languages. This differs from Large Language Models (LLM), such as ChatGTP, which are trained using a broader database of all kinds of text data from across the internet. As a result of their different databases, NMTs are more accurate at translation and LLMs are better at generating new text.
As NMT technologies have become more widely available through online machine translation services such as DeepL, publishers, universities and other translation clients increasingly use them to translate whole documents. They then expect translators to do “machine translation post-editing” (MTPE), cross-referencing the machine translation against the original for a fraction of the price of a normal translation. Of course, in many cases, the translator’s edit of the machine translation is then used to train the machine – known as “human in the loop” translation – apparently moving us closer to a moment in which the human is no longer needed.
Although NMT full text translations have become much more readable, they are still far from being convincingly written by an expert native speaker. At present DeepL even seems to find it hard to do some fairly basic things like cutting sentences in half or reordering them, something which is always necessary in Italian to English translation. This will no doubt improve over time as it begins to identify more complex unwritten grammatical rules within the patterns of the various languages. But for now, to create a text that sounds like it could have been written by a native speaker, a translator will have to change the vast majority of the machine translation, and so it would often be quicker for them to start from scratch, particularly if they are supported by CAT.
Furthermore, a human translator makes numerous creative decisions based on their understanding of the tone, feeling, and sense of the original text, which inevitably also includes deleting bits, rewriting others, and even adding new elements to the text. Of course, the computer could make “creative” decisions like these based on probability in a certain context, perhaps by combining NMT and LLM technologies. But the most probable answer will not always be the best answer, and there is only so much of the context that the computer can take into account without understanding the text. The better it becomes at mimicking a human translator the more decisions like this it will have to make. And the more decisions it makes, the more room there is for error. These errors could either be relatively minor stylistic errors, resulting in a text that feels different to the original, or more serious errors in meaning. And these errors are more likely to be overlooked precisely because the text sounds more convincingly like a native speaker.
For example, the term “il popolo” in Italian would normally be translated as “the people” in English, however, in an article on the workers’ movement the computer translates this Italian sentence “Qual é il motivo per cui il movimento operaio non può avere come soggettività di riferimento il popolo?” as “What is the reason why the workers’ movement cannot have the working class as its reference subjectivity?”. “Il popolo” becomes “the working class” because the computer is smart enough to register that the article is talking about the workers’ movement and the working class is usually around when we’re talking about the workers’ movement. However, this article was specifically about the distinction between “the people” and “the working class”, and so the computer has completely confused the argument. In this case, the problem is precisely the computer’s attempt to take context into account with its “intelligent” non-literal translation. Again, although computers will of course become better at identifying the specificities of a particular context, in order to completely avoid these kinds of mistakes they would have to stop working with probability and instead understand the text they are translating, something which the current technology can only dream of.
Of course you could say that the whole point of post-editing machine translations is to correct errors such as these. But because the translator is engaging with the original through the lens of the computer translation, they might well miss mistakes that they would never have missed had they engaged with the original from the start. The computer translation will also sway their reading of the original in other ways, affecting their ability to engage directly with its tone, nuances and style. Not to mention the fact that the lower pay for this type of translation will mean the translator is more likely to be rushing through it, and so making errors themselves.
What’s more, machine translated texts are also increasingly edited by people who have not seen the original text. In fact, even though I am primarily a translator, I am increasingly asked to edit English texts, and these are invariably machine translations of an Italian original that I am not shown (universities pay less than half as much for an edit as for a translation). In these cases, I do not know what was in the original and so, for example, would not know that working class was originally popolo. As a result, not only will the tone and style suffer, but there is no guarantee that the article will correspond to the meaning of the original. I also spend a lot of time writing comments querying the meaning of computer-translated phrases, and so although I can edit more quickly than I translate, I always end up being paid less per hour, the process is much more frustrating, and the final product is always inferior. Thus, unsurprisingly, machine translation leads to an increase in the quantity of translations and a decrease in their quality, something which seems to be a theme across all AI output (coding, film scripts, educational programmes, etc).
Artificial intelligentsia
When I am sent computer-translated texts, it is often clear that the author has not even read it before sending it to me. In such cases, I can only assume that they are not particularly interested in the content or style of their article, but simply need to get another English publication on their CV. In fact, long before AI was a threat, quality was sacrificed for quantity in much of the academic arts and social sciences, such that it does not seem unrealistic to assume that in some not too distant future computers would be able to produce texts that meet or even exceed the quality of many academic texts in these fields (similar arguments could be made for Netflix dramas). For, with the pressure to write as much as possible, many academics have long abandoned any deep, complex or critical thought that applies to anything in the real world, instead producing texts that are entirely self-referential, regurgitate existing texts and repeat jargon that everyone has forgotten the meaning of: the sort of texts that computers are very good at writing.
Furthermore, in my experience, the content of academic articles is also increasingly impoverished as a result of ring-fenced funding streams. For instance, the research money given to Italy by the EU after COVID requires that academics propose projects related to a limited number of themes, including things like sustainability and artificial intelligence. This leads to Frankenstein’s monster type projects on things like “Early modern monks and AI” or “The Hegelian dialectics of climate change”. The only explanation I can find for projects such as these is that they serve to keep alive an academic elite to give legitimacy to the policies of those funding them.
So if there is already so little “human” left in the academic loop, who cares if further meaning is lost in an AI translation? In fact, why not just fund academics to lend their names to numerous articles written, translated, peer reviewed, published, read, summarised, referenced, edited, rewritten, republished, reread etc by computers on whatever theme funding bodies propose? The computers could even automatically add the publications to the academics’ CVs.
Conclusion
At the moment, MTPE saves little time and produces lower quality translation. While machine translations will improve over time, the arbitrary decisions that AI translation will always have to make is a huge risk for any writer that cares about the content of what they have written, whether that be critical, technical or literary. For this reason, most translators of such texts will continue to use CAT as a sort of super complex interactive dictionary, but will never be completely replaced by computers. However, many translators will be made redundant as low quality and low stakes texts will increasingly be translated by machines, including huge numbers of poor quality academic texts that no-one reads anyway. At least that way I won’t have to read them either.
Footnotes
[1]
Hélène Pielmeier and Paul Daniel O’Mara, The State of the Linguistics Supply Chain: Translators and Interpreters in 2020 (CSA Research, 2020). https://insights.csa-research.com/reportaction/305013106/Toc?SearchTerms=State%20of%20the%20Linguistic%20Supply%20Chain%202020
[2]
Gökhan Fırat, Joanna Gough and Joss Moorken, “Translators in the Platform Economy: A decent work perspective”, Perspectives, 32, No. 3 (2024): 422–440. https://www.tandfonline.com/doi/epdf/10.1080/0907676X.2024.2323213?needAccess=true
[3]
Attila Piróth and Mona Baker, “The Ethics of Volunteerism in Translation: Translators Without Borders and the Platform Economy”, (2019). http://www.pirothattila.com/Piroth-Baker_The_Ethics_of_Volunteerism_in_Translation.pdf